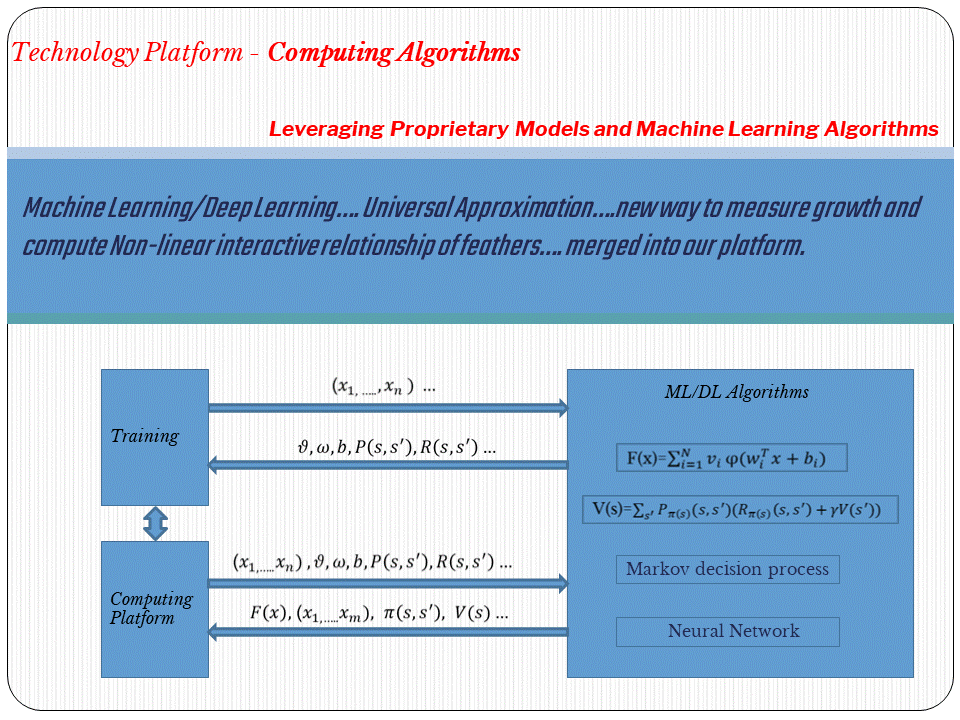
The reason is that the population, based on which we statistically obtain the parameters/models, is often not the same one, based on which we apply them to make projections. In many cases, even if the changes in the two populations are not as significantly seeable as in subprime crisis or in covid-19 pandemic, the projection in the changed population will still be likely to sway away from the range suggested in previous empirical tests.
Therefore, in order to increase the accuracy when applying statistic methods, identifying and measuring the compatibility of populations becomes the "must-have" procedure. Ideally, we want to find identical populations by firstly segmenting historical data and then repeatedly comparing the key parameters in each segment with what we are trying to project. However, all above can work only when our data has a long-enough time span and when we know what parameters to focus on. It is obviously very difficult to meet both pre-conditions, especially for the later.
This is why we have been working on #machinelearning -based method, which allows us to more efficiently identify the changes in data distribution than the statistic method does.